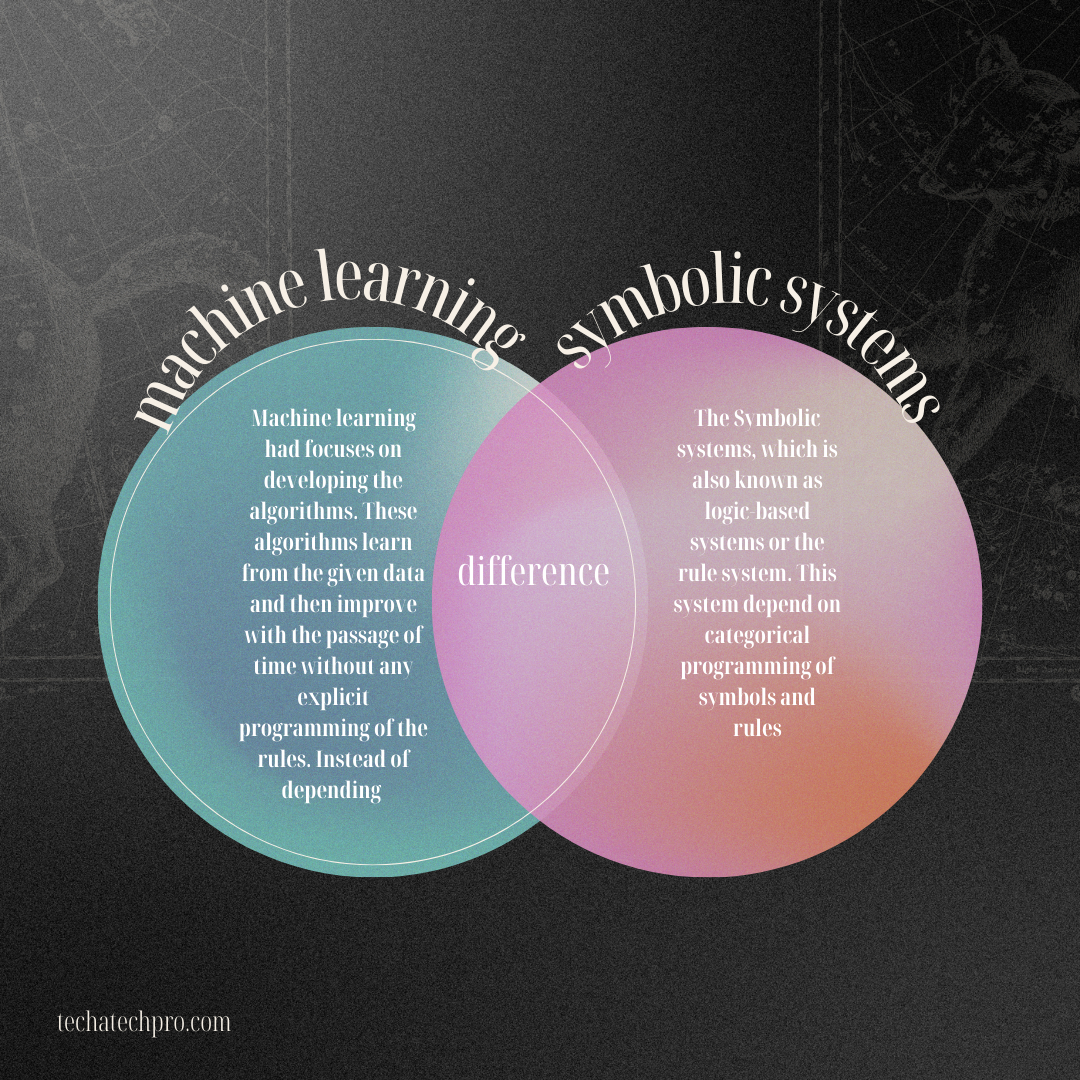
There are the two fundamental approaches in the world of artificial intelligence which are s
Introduction
There are the two fundamental approaches in the world of artificial intelligence which are symbolic systems and machine learning. These two approaches are used to solve the problems. Whereas the main objective of this is to exchange the intelligence which the human beings have. They have the diversity in the different applications and principles. This article helps to understand the differences between the symbolic systems and machine learning, and as well as exploring their unique features and practical implications.
Understanding Symbolic Systems
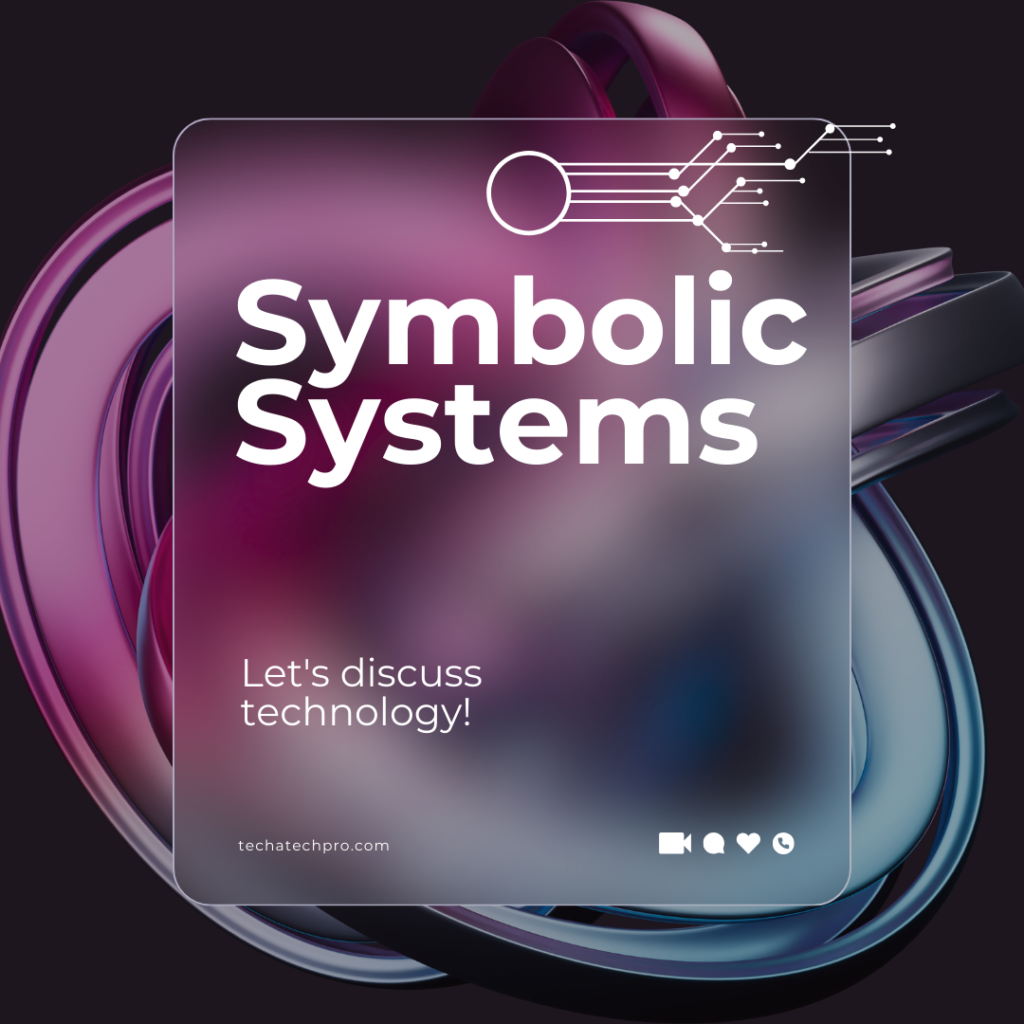
Definition and Concept
The Symbolic systems, which is also known as logic-based systems or the rule system. This system depend on categorical programming of symbols and rules. This programming help us to process the acquired information and make the decisions. These systems work on a already present and well defined set of logical rules. These rules are sometime shows by using the formal logic and as well as symbolic representations.
Logic-Based Approach
Symbolic systems are excellent in performing the tasks that need the categorically reasoning and have the logical deductions. They have the structured approach to solve the problem where knowledge is embedded into a definite set of rules and relationships that help to make the decision.
Rule-Based Systems
In Rule based systems there is set of conditional statements these statements consist of if or then rules. These rules help to give a conclusions which based on given inputs and get them results. These systems are firm and mostly include the well expert knowledge and domain specific rules and regulations.
techatechpro click hear for more information.
Exploring Machine Learning
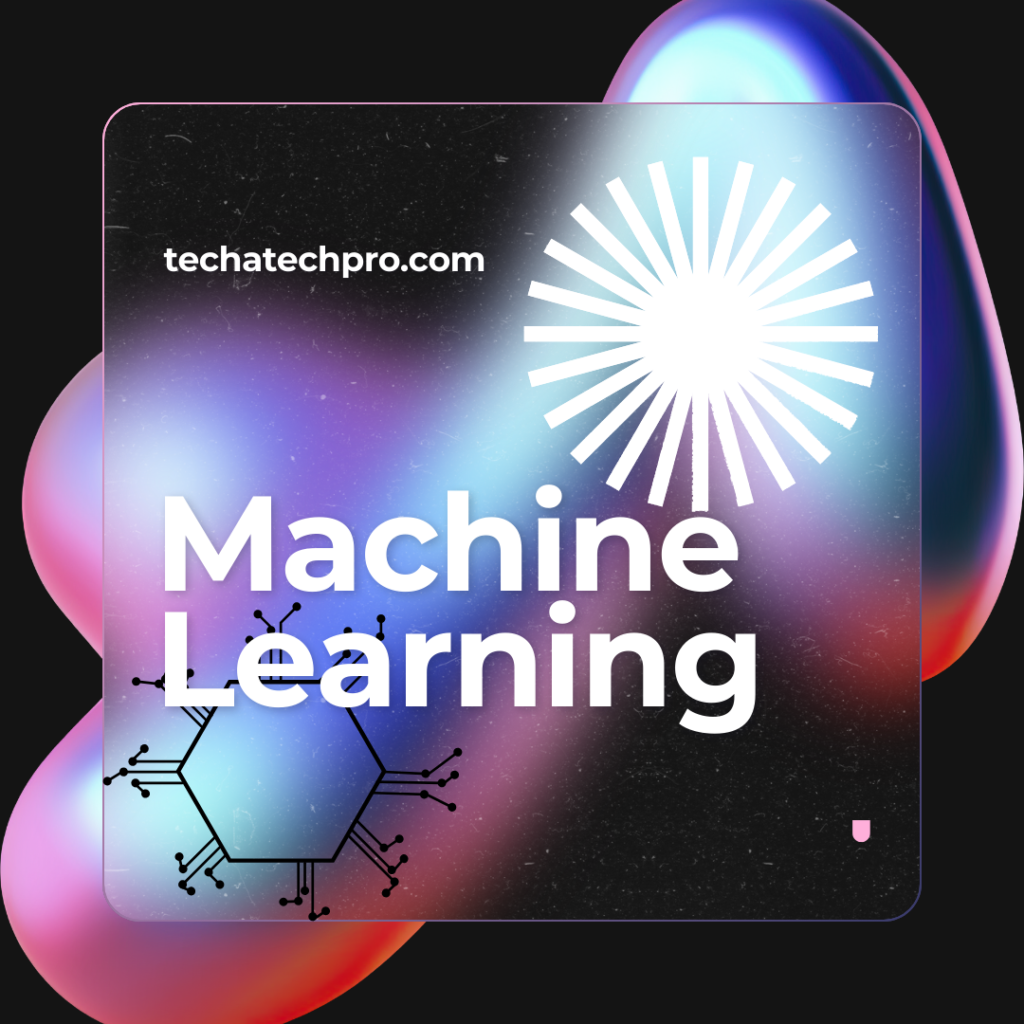
Definition and Basics
Machine learning had focuses on developing the algorithms. These algorithms learn from the given data and then improve with the passage of time without any explicit programming of the rules. Instead of depending on the predefined logic rules, machine learning models also extract the style, patterns and whatever insights from the data through statistical methods and techniques.
Training Models with Data
Basically these Machine learning models are well trained. These models using the large amount of datasets to recognize the patterns and then helps to make the predictions or decisions independently. This process have the learning from the given examples and the adjusting model parameters to reduce the chances of errors.
Types of Machine Learning
Machine learning consists of different techniques, which include the supervised learning, unsupervised learning, and reinforcement learning. These learning techniques work on different ways and pattern.
Key Differences between Symbolic Systems and Machine Learning.
Conceptual Approach
The most basic difference is between the symbolic and machine learning is conceptual approach. The symbolic systems basically depend on predefined rules and regulations and have the categorically representations of knowledge, while machine learning just focus on learning from already given data and then adapting to the patterns.
Adaptability to Data
Symbolic systems are most operative for performing the tasks within the well-defined rules and structured data. But the symbolic systems also have the problem by struggling with handling the bogus information, any type of ambiguity or any unstructured corrupt information. Whereas machine learning are well advanced to handle the complex, diverse and any type of data which are constantly generate.
Handling Complexity
Machine learning is well-suitable for the large scale data and as well as for the complex data which are difficult to solve by using the symbolic system logic alone. It can shows the complicated patterns which does not show through the rule-based systems.
Applications and Use Cases
Symbolic Systems Applications
The Symbolic systems are basically used and applied in expert systems. These systems have the natural language understanding, automated reasoning, and knowledge-based systems. These have the essential explicit instructions and logical conclusions.
Machine Learning Applications
Machine learning mostly have the applications related in image and autonomous vehicles, healthcare diagnostics, speech acknowledgement, recommendation systems, and fraud detection, and have leveraging its aptitude to seek knowledge from a large amounts of data.
Challenges and Limitations
Symbolic Systems Challenges
Symbolic systems face many difficulties and challenges such as in handling the data uncertainty principles, and also context-dependent knowledge, and as well as adapting to vibrant environments where rules may not cover all the aspects.
Machine Learning Limitations
Machine learning need the trained data before to given the output or handling of any type of data. Interpretability of multifarious models become a challenge for the machine learning.
Integration and Future Outlook
The future of AI present in fusion approaches which have the both symbolic systems and machine learning. Symbolic systems and machine learning both work for the well-defined results.
Conclusion
In the bottom line, the major modification between symbolic systems and machine learning are their approach related to problem-solving: plain rule-based logic system versus data-driven learning. Each method are vary in advantages and have limitations. These help to shape the applications among the various domains of AI research and development technology.
Nice article….was very helpful for clear my concepts ❤️ thankyou
Thank you, sir,